Health
New Gene Therapy Approach Offers Precision in Treating Genetic Disorders
This innovation could pave the way for safer, more effective gene therapies for conditions like Fragile X syndrome and Friedreich’s ataxia.
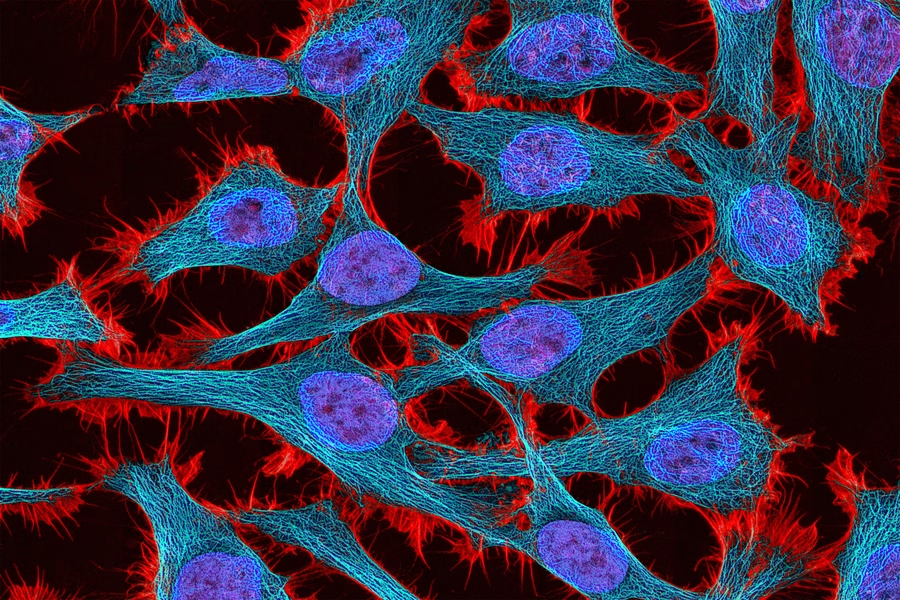
Researchers at MIT have made a significant advancement in gene therapy, offering new hope for treating genetic diseases caused by a missing or defective gene. For years, scientists have pursued gene therapy as a potential cure for a range of monogenic diseases, where a single defective gene causes the disorder. However, the challenge has always been controlling how much of the therapeutic gene is expressed in target cells—too little expression means the therapy won’t work, while too much could result in harmful side effects.
In a study published in Cell Systems, MIT engineers have developed a system that delivers precise control over gene expression levels. Their method, called the ComMAND circuit (Compact microRNA-mediated Attenuator of Noise and Dosage), uses a novel feedback mechanism to regulate the amount of gene product produced in cells. This innovation could pave the way for safer, more effective gene therapies for conditions like Fragile X syndrome and Friedreich’s ataxia.
Led by MIT graduate student Kasey Love and senior author Katie Galloway, a professor in biomedical and chemical engineering, the research focuses on a special type of genetic control circuit known as an incoherent feedforward loop (IFFL). The circuit works by simultaneously activating both the target gene and a microRNA that suppresses the gene’s expression. This self-regulation helps keep gene expression at optimal levels—enough to be effective, but not so much as to cause toxicity.
“Gene supplementation can solve many monogenic disorders if we can control the therapy precisely,” explains Galloway. The team demonstrated this technique by targeting genes associated with Fragile X syndrome and Friedreich’s ataxia—both of which result in neurological and developmental issues. They successfully fine-tuned gene expression to levels that were eight times higher than normal, avoiding the excessive expression seen in earlier gene therapies that could have harmful consequences.
The key advantage of the ComMAND circuit is its compact design, which allows it to be delivered using common viral vectors like lentiviruses or adeno-associated viruses—the same delivery systems used in current gene therapy treatments. This simplicity improves the manufacturability and scalability of the therapy.
While the researchers have demonstrated success in human cells, they acknowledge that further tests in animal models are needed to fine-tune the system for clinical use. They hope this technology could eventually benefit patients with a range of genetic disorders, including muscular dystrophy, spinal muscular atrophy, and Rett syndrome.
“Despite the small patient populations for many of these rare diseases, we are working to develop tools that are robust enough for widespread use,” says Galloway, emphasizing the importance of such innovations in addressing diseases that often lack funding and research attention due to their rarity.
The breakthrough is a promising step toward making gene therapy not just a theoretical cure but a reliable, safe treatment option for genetic disorders, with potential applications that could transform the landscape of precision medicine in the years to come.
Health
PUPS – the AI tool that can predict where exactly proteins are in human cells
Dubbed, the Prediction of Unseen Proteins’ Subcellular Localization (or PUPS), the AI tool can account for the effects of protein mutations and cellular stress—key factors in disease progression.
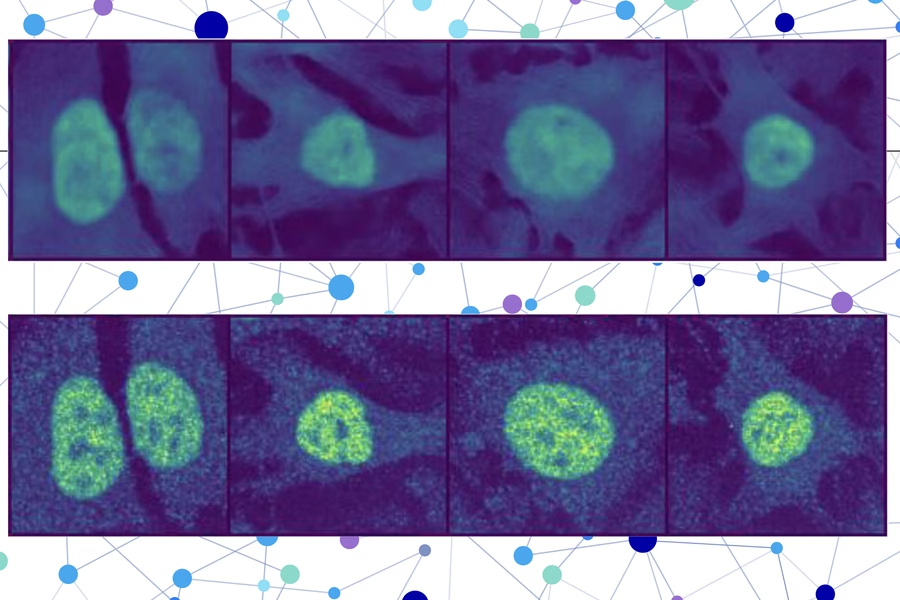
Researchers from MIT, Harvard University, and the Broad Institute have unveiled a groundbreaking artificial intelligence tool that can accurately predict where proteins are located within any human cell, even if both the protein and cell line have never been studied before. The method – Prediction of Unseen Proteins’ Subcellular Localization (or PUPS) – marks a major advancement in biological research and could significantly streamline disease diagnosis and drug discovery.
Protein localization—the precise location of a protein within a cell—is key to understanding its function. Misplaced proteins are known to contribute to diseases like Alzheimer’s, cystic fibrosis, and cancer. However, identifying protein locations manually is expensive and slow, particularly given the vast number of proteins in a single cell.
The new technique leverages a protein language model and a sophisticated computer vision system. It produces a detailed image that highlights where the protein is likely to be located at the single-cell level, offering far more precise insights than many existing models, which average results across all cells of a given type.
“You could do these protein-localization experiments on a computer without having to touch any lab bench, hopefully saving yourself months of effort. While you would still need to verify the prediction, this technique could act like an initial screening of what to test for experimentally,” said Yitong Tseo, a graduate student in MIT’s Computational and Systems Biology program and co-lead author of the study, in a media statement.
Tseo’s co-lead author, Xinyi Zhang, emphasized the model’s ability to generalize: “Most other methods usually require you to have a stain of the protein first, so you’ve already seen it in your training data. Our approach is unique in that it can generalize across proteins and cell lines at the same time,” she said in a media statement.
PUPS was validated through laboratory experiments and shown to outperform baseline AI methods in predicting protein locations with greater accuracy. The tool is also capable of accounting for the effects of protein mutations and cellular stress—key factors in disease progression.
Published in Nature Methods, the research was led by senior authors Fei Chen of Harvard and the Broad Institute, and Caroline Uhler, the Andrew and Erna Viterbi Professor at MIT. Future goals include enabling PUPS to analyze protein interactions and make predictions in live human tissue rather than cultured cells.
Health
Robot Helps Elderly Sit, Stand, and Stay Safe from Falls
The innovation comes at a time when the United States faces a dramatic demographic shift
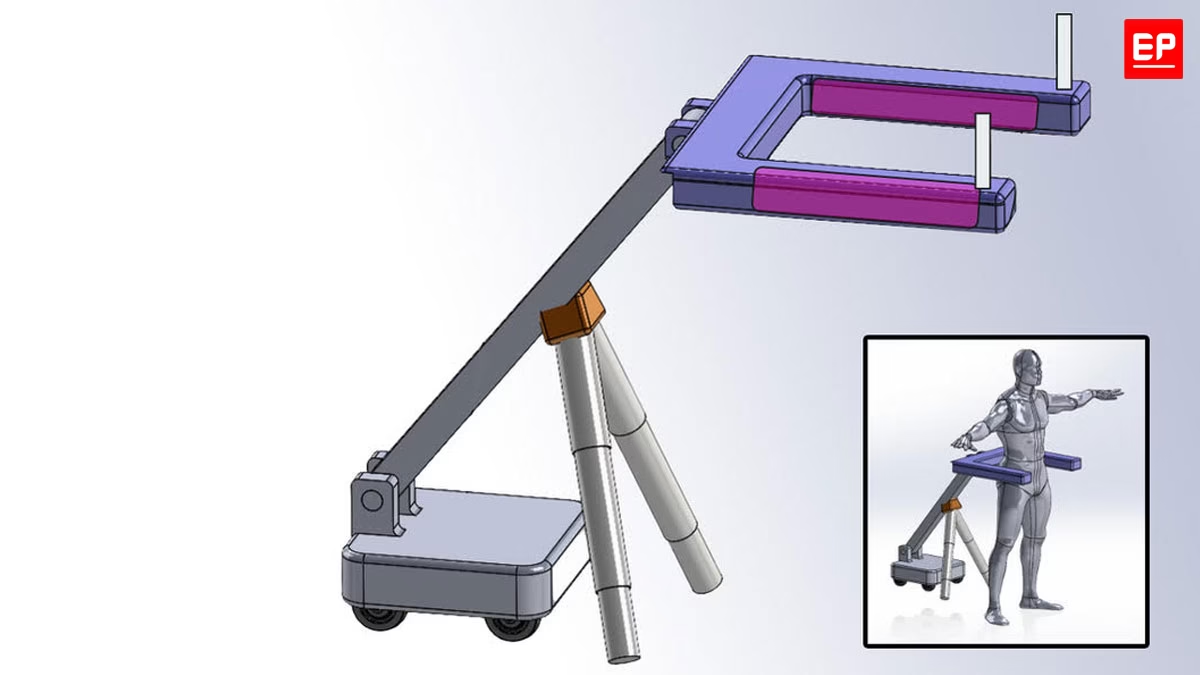
As America’s population ages faster than ever before, a team of engineers at MIT is turning to robotics to meet the growing eldercare crisis. Their latest invention, the Elderly Bodily Assistance Robot—or E-BAR—aims to provide critical physical support to seniors navigating life at home, potentially reducing the risk of injury and relieving pressure on a strained care system.
The innovation comes at a time when the United States faces a dramatic demographic shift. The nation’s median age has climbed to 38.9, nearly ten years older than in 1980. By 2050, the number of adults over 65 is projected to surge from 58 million to 82 million. As demand for care rises, the country is simultaneously grappling with shortages in care workers, escalating healthcare costs, and evolving family structures that leave many elderly adults without daily support.
“Eldercare is the next great challenge,” said Roberto Bolli, a graduate student in MIT’s Department of Mechanical Engineering and one of E-BAR’s lead designers, in a media statement. “All the demographic trends point to a shortage of caregivers, a surplus of elderly persons, and a strong desire for elderly persons to age in place.”
E-BAR is designed to address exactly that challenge. The mobile robot acts as a robotic support system, following a user from behind and offering both steadying handlebars and rapid intervention in case of a fall. It can support a person’s full weight and includes side airbags that inflate instantly to catch users if they begin to fall—without requiring them to wear any equipment or harnesses.
“Many older adults underestimate the risk of fall and refuse to use physical aids, which are cumbersome, while others overestimate the risk and may not exercise, leading to declining mobility,” said Harry Asada, the Ford Professor of Engineering at MIT, in a media statement. “Our design concept is to provide older adults having balance impairment with robotic handlebars for stabilizing their body. The handlebars go anywhere and provide support anytime, whenever they need.”
The robot consists of a heavy, 220-pound base equipped with omnidirectional wheels, allowing it to maneuver easily through typical home spaces. From its base, articulated bars extend and adjust to assist users in standing or sitting, and the handlebars provide a natural, unrestrictive grip. In testing, E-BAR successfully helped an older adult complete everyday movements such as bending, reaching, and even stepping over the edge of a bathtub.
“Seeing the technology used in real-life scenarios is really exciting,” said Bolli.
The team’s design, which will be presented later this month at the IEEE Conference on Robotics and Automation (ICRA), aims to eliminate the physical constraints and stigmas often associated with eldercare devices. Their approach prioritizes both independence and safety—key values for aging Americans seeking to remain in their homes longer.
While E-BAR currently operates via remote control, the team plans to add autonomous capabilities and streamline the device’s design for home and facility use. The researchers are also exploring ways to integrate fall-prediction algorithms, developed in a parallel project in Asada’s lab, to adapt robotic responses based on a user’s real-time risk level.
“Eldercare conditions can change every few weeks or months,” Asada noted. “We’d like to provide continuous and seamless support as a person’s disability or mobility changes with age.”
As the nation prepares for the realities of an aging population, MIT’s work offers a glimpse into a future where robotics play a central role in eldercare—enhancing both quality of life and personal dignity for millions of older adults.
Health
Scientist urges need for an Indian-specific blood parameter reference range
In India, standard blood parameter reference ranges aren’t representative of the local population; but based on conclusions derived from population studies in the West.
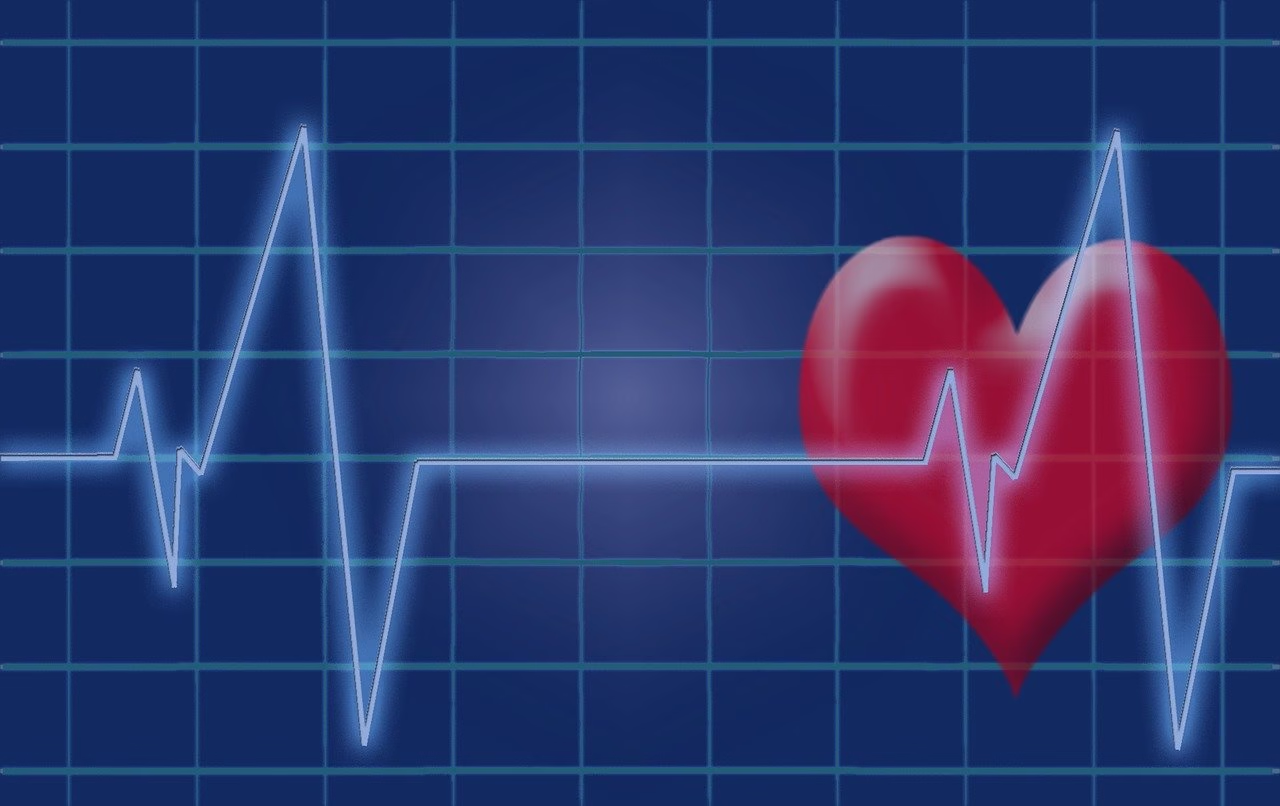
Prof. Ullas Kolthur-Seetharam, a leading Indian scientist in metabolism and aging, has urged for the re-optimization of standard blood parameter reference ranges to better suit Indian populations, highlighting that current values are based on Western populations and may not account for India-specific factors.
Speaking at the National Technology Day (NTD) 2025 lecture at the Biotechnology Research and Innovation Council-Rajiv Gandhi Centre for Biotechnology (BRIC-R caballoGCB), Kerala, India, Prof. Kolthur-Seetharam emphasized the need for tailored diagnostic benchmarks to improve the accuracy of diagnosing metabolic disorders like diabetes and cardiovascular diseases in India.
“Genetic, dietary, and environmental differences can significantly alter biomarkers,” said Prof. Kolthur-Seetharam, Director of the Centre for DNA Fingerprinting and Diagnostics (BRIC-CDFD), Hyderabad, India. He noted that emerging research reveals how dietary patterns influence health through mitochondrial function and epigenetic regulation, necessitating India-specific reference ranges.
Currently on deputation from the Tata Institute of Fundamental Research (TIFR), Mumbai, Prof. Kolthur-Seetharam has made significant contributions to understanding the interplay of mitochondrial function, epigenetics, and nutrition in shaping health and longevity. He also founded The Advanced Research Unit on Metabolism, Development & Aging (ARUMDA) at TIFR, a pioneering initiative tackling India’s challenges with malnutrition, non-communicable diseases, and aging through interdisciplinary research.
-
Earth4 months ago
How IIT Kanpur is Paving the Way for a Solar-Powered Future in India’s Energy Transition
-
Space & Physics3 months ago
Could dark energy be a trick played by time?
-
Society4 months ago
Starliner crew challenge rhetoric, says they were never “stranded”
-
Earth2 months ago
122 Forests, 3.2 Million Trees: How One Man Built the World’s Largest Miyawaki Forest
-
Space & Physics3 months ago
Sunita Williams aged less in space due to time dilation
-
Space & Physics6 months ago
Obituary: R. Chidambaram, Eminent Physicist and Architect of India’s Nuclear Program
-
Society4 months ago
Sustainable Farming: The Microgreens Model from Kerala, South India
-
Women In Science3 months ago
Neena Gupta: Shaping the Future of Algebraic Geometry