Society
Here’s a technique to assess the reliability of AI models before it’s deployed
“All models can be wrong, but models that know when they are wrong are more useful.”
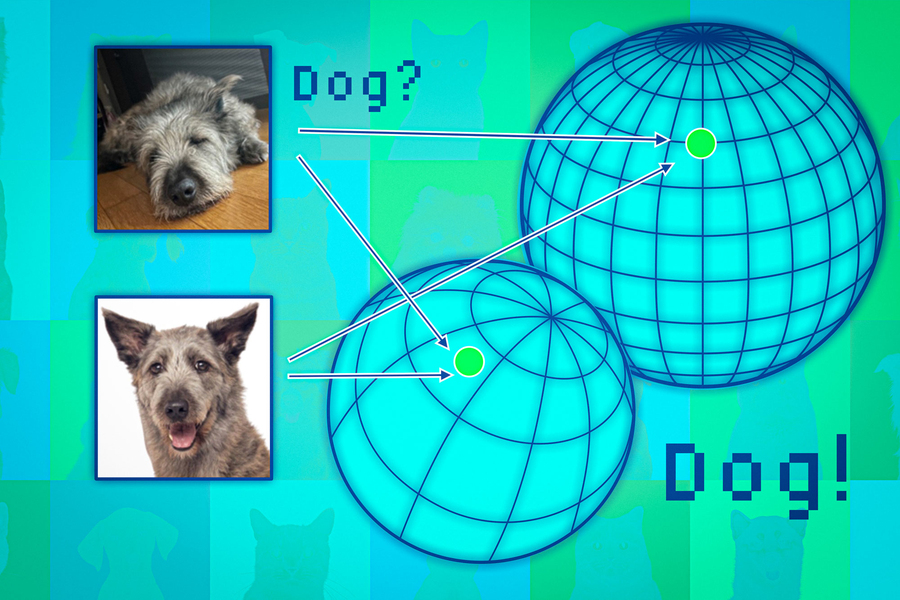
MIT researchers, together with the MIT-IBM Watson AI Lab, have designed a method to assess the dependability of foundational AI models prior to their deployment in specific tasks.
Their method involves analyzing a set of slightly varied models and evaluating how consistently each model learns representations of the same test data point. Consistent representations indicate a reliable model.
This technique offers significant practical implications. It enables decision-makers to determine whether a AI model is suitable for deployment in specific settings without the necessity of testing it on real-world datasets
When compared against current advanced methods, their technique outperformed others in accurately measuring the reliability of foundation models across various downstream classification tasks.
This technique offers significant practical implications. It enables decision-makers to determine whether a AI model is suitable for deployment in specific settings without the necessity of testing it on real-world datasets. This capability is particularly valuable in contexts where accessing datasets is restricted due to privacy concerns, such as in healthcare settings. Furthermore, the method facilitates the ranking of models based on their reliability scores, empowering users to select the most appropriate model for their intended task.
Navid Azizan, senior author of the study and Esther and Harold E. Edgerton Assistant Professor in the MIT Department of Mechanical Engineering and the Institute for Data, Systems, and Society (IDSS), highlights the importance of models being aware of their own limitations: “All models can be wrong, but models that know when they are wrong are more useful.” He emphasizes the challenge in quantifying uncertainty or reliability for foundation models due to their abstract representations, which are inherently difficult to compare. Azizan concludes that their method offers a means to precisely quantify how reliable a model’s representation is for any given input data.
The research paper, authored by Young-Jin Park, a graduate student at LIDS; Hao Wang, a research scientist at the MIT-IBM Watson AI Lab; and Shervin Ardeshir, a senior research scientist at Netflix, will be presented at the Conference on Uncertainty in Artificial Intelligence.
Traditional machine-learning models are typically trained for specific tasks, providing definite predictions based on inputs such as determining whether an image features a cat or a dog. In contrast, foundation models are pretrained on generalized data without prior knowledge of the diverse tasks they will eventually tackle. Users fine-tune these models for specific applications after their initial training.
Unlike conventional models that yield straightforward outputs like “cat” or “dog,” foundation models generate abstract representations based on input data. Evaluating the reliability of such models poses a unique challenge. Researchers adopted an ensemble approach by training multiple models with similar characteristics but slight variations.
“Our concept is akin to gauging consensus. If all these foundation models consistently produce similar representations for any given dataset, then we can infer the model’s reliability,” explains Park.
The challenge arose in comparing these abstract representations. He says that these models output vectors, composed of numerical values, making direct comparisons difficult. The solution involved employing a method known as neighborhood consistency.
In their methodology, researchers established a set of dependable reference points to test across the ensemble of models. They examined neighboring points surrounding each model’s representation of the test data point to gauge consistency.
By assessing the coherence among neighboring points, they could effectively estimate the reliability of the models.
Books
The Rise of U.S. Retail Giants: A Century of Political and Economic Shaping
Currently, 90% of Americans live within 10 miles of a Walmart, and five of the top 10 U.S. employers—Walmart, Amazon, Home Depot, Kroger, and Target—are retailers
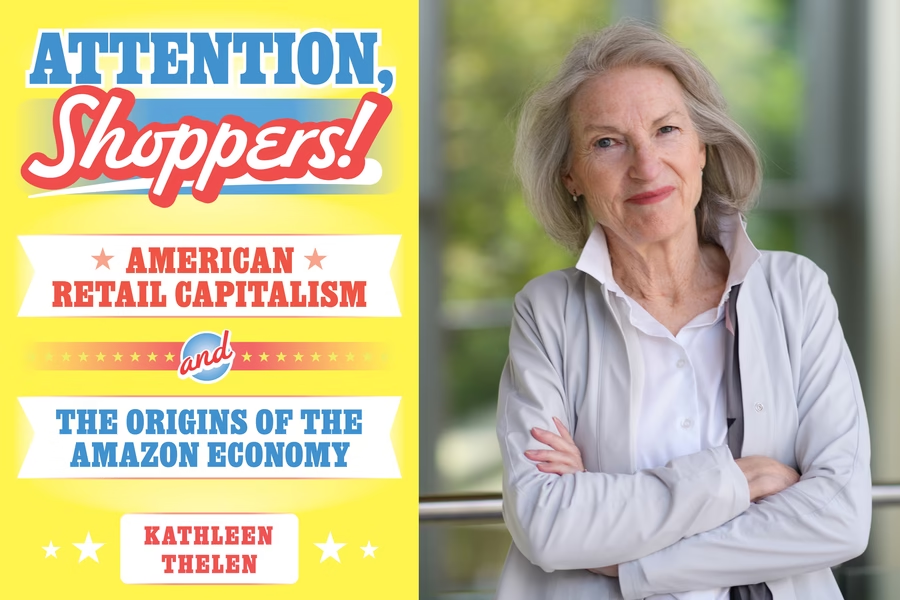
The U.S. retail sector, once dominated by small, independent merchants, has transformed over the past century into a landscape controlled by retail giants. In the late 19th century, most U.S. retail was local. However, this shifted with the rise of catalog retailers like Sears and Roebuck, which saw rapid growth, followed by Montgomery Ward’s expansion. By the 1930s, chain stores began to proliferate, with the Atlantic and Pacific (A&P) supermarkets leading the pack with over 15,000 locations.
Fast-forward to today, and the dominance of retailers like Walmart, Amazon, and Target is undeniable. Currently, 90% of Americans live within 10 miles of a Walmart, and five of the top 10 U.S. employers—Walmart, Amazon, Home Depot, Kroger, and Target—are retailers. In addition, logistics giants UPS and FedEx play a crucial role in supporting the retail economy.
This prevalence of massive retail chains is largely unique to the U.S., where domestic consumption is a driving force behind economic growth. Additionally, the U.S. has five times as much retail space per capita as Japan and the U.K., and 10 times as much as Germany. Unlike in Europe, the U.S. has few regulations limiting shopping hours.
How did we arrive at this point? While major chains like Walmart and Amazon are known for their business prowess, the full story involves over a century of political and legal debates that shaped the landscape of U.S. retailing. MIT political scientist Kathleen Thelen, in her new book Attention, Shoppers! American Retail Capitalism and the Origins of the Amazon Economy, dives into the role of political and legal forces in the rise of large, low-cost retailers.
“The markets that we take as given, that we think of as the natural outcome of supply and demand, are heavily shaped by policy and by politics,” Thelen explains.
Thelen’s book offers a unique perspective, drawing comparisons with European economies and taking a historical approach to the growth of chain retailing. For instance, she highlights how alternative commercial arrangements, like cooperatives, were stifled by U.S. antitrust laws, which favored big corporations while suppressing smaller competitors. This legal framework gave a significant advantage to large retailers, including Sears, which relied on the U.S. Postal Service’s money order system to reach customers who lacked bank accounts.
Smaller retailers resisted the expansion of large chains, particularly during the Great Depression, but big retailers found ways around regulatory constraints. “Antitrust laws in the United States were very forbearing toward big multidivisional corporations and very punitive toward alternative types of arrangements like cooperatives, so big retailers got a real boost in that period,” Thelen says. Over time, antitrust law increasingly prioritized consumer prices, further benefiting low-cost retailers.
As Thelen argues, prioritizing price reduction often leads to lower wages for workers, with large retailers driving down wages both directly and through pressure on suppliers. “If you prioritize prices, one of the main ways to reduce prices is to reduce labor costs,” she says, noting that low-cost discounters are often low-wage employers.
In her analysis, Thelen suggests that the American retail system’s focus on low prices, low wages, and high consumer convenience has led to a “deep equilibrium,” where low-wage workers rely on these retail giants to make ends meet. Meanwhile, the speed of modern delivery systems has become a normal part of American shopping culture.
“The triumph of these types of retailers was not inevitable,” Thelen reflects. “It was a function of politics and political choice.” With ongoing debates about labor law reforms and antitrust enforcement, the current retail equilibrium may persist for the foreseeable future, unless significant changes are made to the system.
Through Attention, Shoppers!, Thelen offers readers a comprehensive look at the economic forces that have shaped the retail sector, helping explain the giant retail landscape many Americans take for granted today.
Society
Jio Joins Forces with SpaceX’s Starlink to Bring High-Speed Internet to India
India’s richest man Mukesh Ambani’s Jio Partners with SpaceX for a Digital Revolution
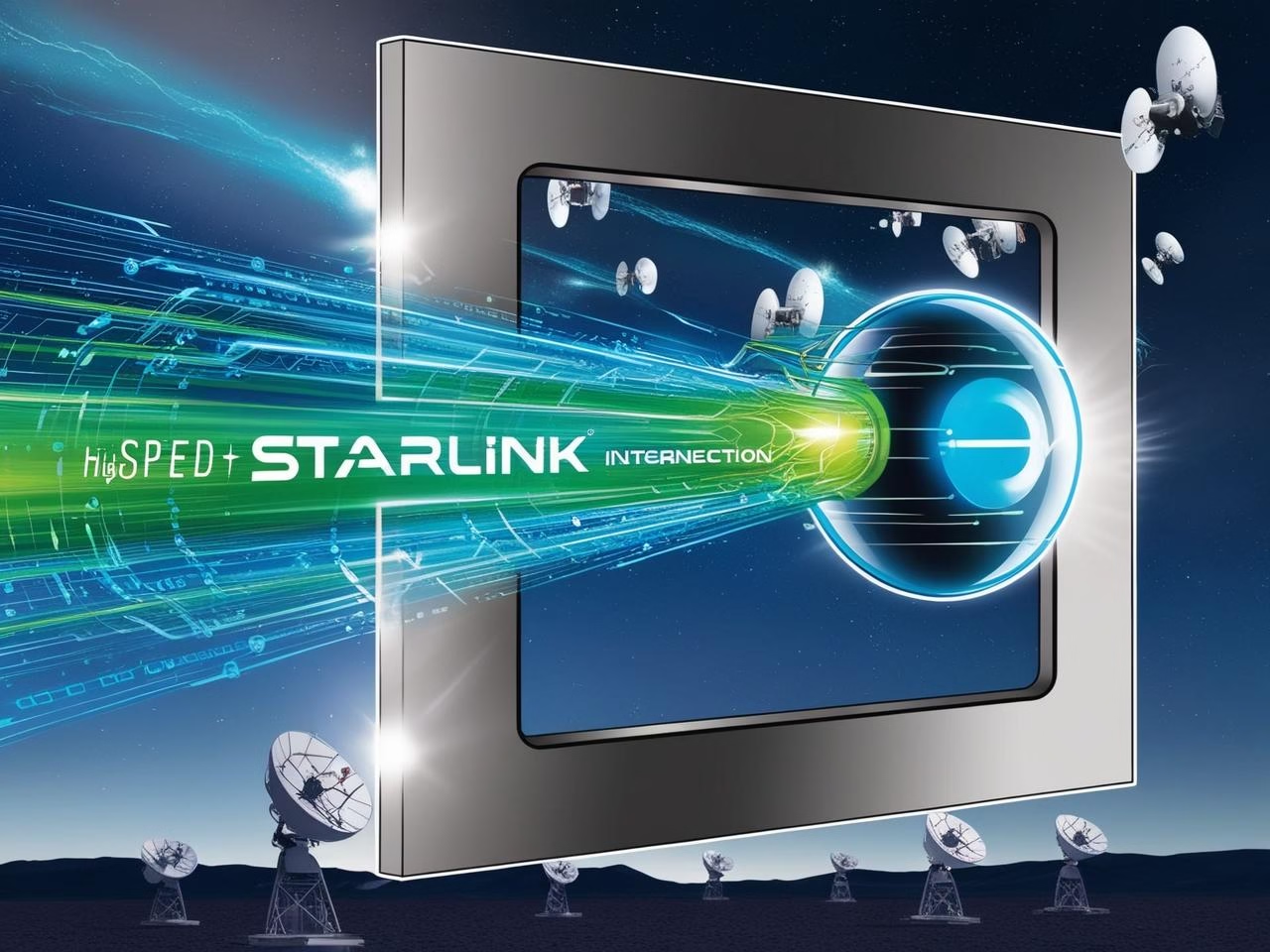
In a groundbreaking move, Jio Platforms Limited (JPL), a subsidiary of India’s Reliance Industries Limited, has announced a strategic partnership with SpaceX to offer Starlink’s high-speed broadband internet services across India. This collaboration comes as part of Jio’s ambition to expand its broadband offerings and transform connectivity in the country, especially in rural and remote areas.
The partnership between Jio, led by India’s richest man, Mukesh Ambani, and SpaceX, led by US billionaire Elon Musk, marks a significant step in bridging the digital divide and accelerating India’s digital ecosystem. By bringing Starlink’s advanced low Earth orbit (LEO) satellite internet into its fold, Jio is positioning itself at the forefront of India’s broadband evolution, promising to provide affordable and high-speed internet to even the most remote corners of the country.
Through this agreement, Jio will integrate Starlink’s services into its vast network, offering them to both consumers and businesses across India. Customers will be able to access Starlink’s solutions through Jio’s retail outlets as well as its online platforms, ensuring a seamless and efficient experience for users nationwide.
“Ensuring that every Indian, no matter where they live, has access to affordable and high-speed broadband remains Jio’s top priority,” said Mathew Oommen, Group CEO of Reliance Jio, in a statement. “Our collaboration with SpaceX to bring Starlink to India strengthens our commitment and marks a transformative step toward seamless broadband connectivity for all. By integrating Starlink into Jio’s broadband ecosystem, we are expanding our reach and enhancing the reliability and accessibility of high-speed broadband in this AI-driven era, empowering communities and businesses across the country.”
Jio’s extensive infrastructure, paired with Starlink’s pioneering satellite technology, will address the connectivity challenges in India’s most underserved areas, ensuring the benefits of the digital age are accessible to all. The collaboration will also allow Jio to complement its existing broadband services, such as JioAirFiber and JioFiber, by providing high-speed internet in hard-to-reach locations more quickly and affordably.
Additionally, Jio and SpaceX are exploring further areas of collaboration, looking for innovative ways to strengthen India’s digital landscape. Gwynne Shotwell, President and Chief Operating Officer of SpaceX, commented, “We applaud Jio’s commitment to advancing India’s connectivity. We are looking forward to working with Jio and receiving authorization from the Government of India to provide more people, organizations, and businesses with access to Starlink’s high-speed internet services.”
In an interesting twist, Jio’s partnership with Starlink comes just one day after India’s second-largest telecom operator, Airtel, also signed a deal with Starlink. This move indicates that India’s telecom sector is witnessing a significant transformation as leading operators race to offer cutting-edge broadband services through satellite technology, further boosting the country’s digital revolution.
As part of its long-term strategy, Jio continues to innovate and diversify its offerings, positioning itself as a leader in the broadband space with cutting-edge solutions. With this collaboration, Jio not only aims to enhance the reach of its broadband services but also solidifies its role in advancing India’s goal of becoming a global leader in the digital economy.
The union of Jio’s expansive infrastructure and SpaceX’s space-based internet promises to accelerate India’s journey toward becoming a digitally connected nation, ensuring that no part of the country is left behind in the fast-evolving digital landscape.
Society
New Research Could Allow People to Correct Robots’ Actions in Real-Time
Through basic interactions like pointing to the object, tracing a path on a screen, or physically nudging the robot’s arm, you could guide it to complete the task more accurately.
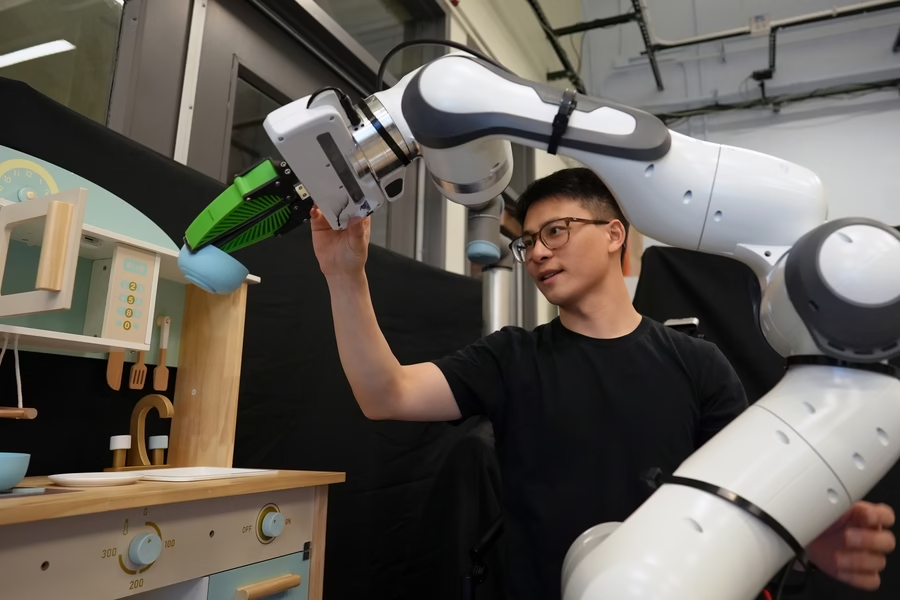
A breakthrough framework developed by researchers from MIT and NVIDIA may soon allow people to correct a robot’s actions in real-time using simple, intuitive feedback—similar to how they would guide another person.
Imagine you’re doing the dishes and a robot grabs a soapy bowl from the sink—but its gripper misses the mark. Instead of having to retrain the robot from scratch, a new method could enable you to fix its behaviour in real time. Through basic interactions like pointing to the object, tracing a path on a screen, or physically nudging the robot’s arm, you could guide it to complete the task more accurately.
This new approach eliminates the need for users to collect data and retrain the robot’s machine-learning model, unlike other traditional methods. Instead, it allows the robot to immediately adjust its actions based on user feedback to get as close as possible to fulfilling the user’s intent.
In tests, the framework’s success rate was 21 percent higher than an alternative method that did not leverage human corrections.
“This approach is designed to let robots perform tasks effectively right out of the box,” says Felix Yanwei Wang, an MIT graduate student in electrical engineering and computer science (EECS) and the lead author of a paper on the framework. “We can’t expect laypeople to gather data and fine-tune models. If a robot doesn’t work as expected, users should have an intuitive way to fix it.”
Wang’s co-authors include Lirui Wang PhD ’24, Yilun Du PhD ’24, senior author Julie Shah, MIT professor of aeronautics and astronautics and director of the Interactive Robotics Group at CSAIL, along with Balakumar Sundaralingam, Xuning Yang, Yu-Wei Chao, Claudia Perez-D’Arpino PhD ’19, and Dieter Fox from NVIDIA. The research will be presented at the upcoming International Conference on Robots and Automation.
A New Approach to Robot Correction
Currently, many robots use generative AI models trained on vast amounts of data to perform tasks. These models can solve complex tasks but often struggle to adapt to real-world situations that differ from their training environment. For example, a robot might fail to pick up a box from a shelf if the shelf in the user’s home is arranged differently than in its training environment.
To address this, engineers often collect new data and retrain the model—a time-consuming and costly process. However, the new MIT-NVIDIA framework allows users to interact with the robot during deployment, correcting its behavior in real time without the need for retraining.
“We want users to guide the robot without causing mistakes that could misalign with their intent,” says Wang. “The goal is to provide feedback that adjusts the robot’s behavior in a way that is both valid and aligned with the user’s goals.”
The system offers three ways for users to provide feedback: they can point to the object they want the robot to interact with, trace a desired trajectory on a screen, or physically nudge the robot’s arm. Wang explains, “Physically nudging the robot is the most direct way to specify user intent without losing any of the information.”
Ensuring Valid Actions
To avoid the robot making invalid moves—like colliding with nearby objects—the researchers developed a sampling procedure. This technique ensures that the robot chooses actions that are both feasible and aligned with the user’s request.
“Rather than just imposing the user’s will, we allow the robot to take the user’s intent into account while ensuring the actions remain valid,” Wang says.
The researchers’ framework outperformed other methods during tests with a real robot arm in a toy kitchen. While the robot might not always complete tasks immediately, the system allows users to correct it on the spot, without waiting for it to finish and then provide new instructions.
The framework also has the potential to learn from user corrections. For instance, if a user nudges the robot to pick up the correct bowl, the robot could log this action and incorporate it into its future behavior, gradually improving over time.
“The key to continuous improvement is having a way for users to interact with the robot,” says Wang. “This method makes that possible.”
Looking ahead, the researchers aim to improve the speed of the sampling procedure and test the framework in new, more complex environments, paving the way for robots that are more adaptable to real-world scenarios.
-
EDUNEWS & VIEWS5 months ago
India: Big Science in the 20th century and beyond
-
Interviews6 months ago
Memory Formation Unveiled: An Interview with Sajikumar Sreedharan
-
Society6 months ago
Global tech alliance: Nvidia partners with Reliance to transform AI landscape in India
-
The Sciences6 months ago
Prof Saleem Badat awarded ASSAf Science-for-Society Gold Medal
-
Earth5 months ago
The wildfires, floods, and heatwaves: Understanding the science behind climate change
-
Society6 months ago
Do not compete the competition
-
Space & Physics5 months ago
How Shyam Gollakota is revolutionizing mobile systems and healthcare with technology
-
Space & Physics5 months ago
Chandrayaan-3: The moon may have had a fiery past